Fighting Healthcare Disparities | Equitable and Accessible Healthcare for All
- 0.5
- 1
- 1.25
- 1.5
- 1.75
- 2
Paul Anderson: Welcome to Smart Healthcare Safety from ECRI, the most trusted voice in healthcare committed to advancing effective evidence- based care. I'm your host, Paul Anderson, and for more than 10 years I've overseen our Patient Safety, Risk and Quality membership programs here at ECRI. Tens of thousands of healthcare leaders rely on us as an independent, trusted authority to improve the safety, quality and cost effectiveness of care across all healthcare settings worldwide. You can learn more about our unique capabilities to improve outcomes at www. ecri. org. Today's episode is part of a series we're recording for the ECRI and the ISNP Patient Safety Organization's deep dive report. This year's deep dive focuses on issues of racial and ethnic disparities in care, and we're talking to PSO members and others to hear about their initiatives to fight these disparities. Our first guest is from Parkland Health& Hospital System, one of the country's largest and most progressive safety net hospitals.
Brett Moran: Hello, my name is Brett Moran. I'm the senior vice president and chief medical information officer at Parkland. I'm also a associate chief medical officer, and I'm over all things clinical informatics.
Paul Anderson: Our second guest is from Parkland Center for Clinical Innovation, a leading nonprofit data science, artificial intelligence, and innovation organization affiliated with Parkland Health& Hospital System.
Steve Miff: Hello, everybody. Steve Miff, I am the president and CEO at PCCI, the Parkland Center for Clinical Innovation.
Paul Anderson: Dr. Moran, I mentioned that Parkland is in the Dallas area. Can you tell us a little bit more about the health system and the community that you serve?
Brett Moran: Yeah, sure. So Parkland is one of the last remaining county safety net hospitals. We were founded in 1894. We're licensed for about 880 beds, and we serve the patients of the county. We have a lot of charity care. Over 33% of our patients that present have charity. Another 29% are Medicaid, and another 18% are Medicare. We have some interesting facts. One in four physicians practicing in Dallas today trained at Parkland, and one in every 250 babies born in Texas were born at Parkland.
Paul Anderson: That really speaks to impact, right, to have that proportion of the physicians in the county to have been gone through Parkland.
Brett Moran: Absolutely.
Paul Anderson: That's a huge reach. Yeah. And then, Dr. Miff, can you tell us a little bit more about what PCCI does? Particularly, we want to get into a little bit the community health needs assessment efforts.
Steve Miff: Absolutely. PCCO is set up as a nonprofit. The core of it, too, we are a data science and social determinants of health innovation hub. As mentioned, affiliated with Parkland. Our main focus is on bringing together clinical information with information about somebody's life and applying advanced data science methods to better understand an individual, and focusing our work on this innovation in the digital health technology in advanced analytics to drive health equity. We're focusing on, how do we optimize access using these analytics for vulnerable populations? So at the core of it, we're trying to use data to better understand an individual. Understand not only their clinical needs but their life, and trying to do that at scale and tee that up for frontline staff and for individuals themselves so that care can be done in a proactive way, in a way that's all encompassing.
Paul Anderson: So Dr. Moran, we're recording this in early 2022, kind of very end of February. We've been at this pandemic thing now for coming up on two years. Can you take us back to the beginning of that, to the winter or early spring of 2020? What was the experience at Parkland at the beginning of the pandemic?
Brett Moran: Yeah. Yeah. It's funny trying to think about it now, because it feels like it was so long ago. I don't have to describe when the first cases were described in Wuhan, China in December of 2019. But the minute that happened, and certainly by January we were having conversations about this. By late February of 2020, Parkland had declared its own official disaster. By the beginning of March, we had created our own COVID Command Center. We did not have our first cases till the middle of March. And from then, things were really rapid paced. We had our COVID Command Center. We started working with Steve's team creating a dashboard for COVID and also trying to scramble to create some predictive analytics about what's going to happen with COVID. How big is the wave going to be? Can we even handle that? But we also did a lot of interesting things. We were one of the first healthcare organizations in the country to create a discreet tactical care unit for managing COVID cases within a complete negative air pressure isolation unit. We scrambled to create these Frankenstein, we called them Molly machines that were basically webcams on wheels that would plug into the LAN port. We would put them in patient rooms, and it would allow us to pan and tilt and have conversations with patients and monitor them without people having to go into the rooms. We created dozens of those for the hospital. We started having multidisciplinary, concurrent, virtual rounding in the ICUs. So the ICU team and then the nurse would have all of these specialists remote end virtual, have a Zoom meeting and sit there at the same time and do rounding virtually on a patient. That was something that they hadn't done before, and they really loved it. They've actually continued that habit since then. We stood up drive- through testing. We had massive expansion of virtual visits just like everybody else, over a 900% increase. And then we began doing a lot of collaborations with Steve and his team, as I said, with the COVID predictions, and then we started working with the county health department doing semi- automated contact tracing. We set up a solution for them, and then we started trying to figure out what are some other things we can do to try to help.
Paul Anderson: Can you tell me a little bit more about those predictive analytics? If you can, without going too, too deep. What were you trying to take in and consume? And then what were you trying to do with that to spit back out to assess? We you assessing the risk of the individual patients, the population's? What was the goal there?
Brett Moran: What we were trying to assess was for the predictive analytics, at the beginning of the predictive analytics was how big was the wave going to be of patients coming in with COVID to Parkland? And was that something that we would have enough hospital beds or ICU beds to handle? It was unfortunate at the time, and probably even now still, is that there is not a great single point of information to go in this country to say," Help me figure out how my health system can withstand this next wave, the Omicron, the Delta." So we had to figure it out ourselves, and Steve and his team helped us with that. We used best practices. We used what some other health systems were doing. We did a lot of," Let's find someone that looks like us, that's a few weeks ahead of us, and let's see what they're doing." And that was how we did it. And then after we had gotten those predictions, it was a little bit of wait and watch, and hope and pray that it doesn't get to be really ugly, and that we're right or that we overestimated it. But it was during that time that we then started saying," Well, what else can we do? What are some other things that we can do using some of the tools we have to help the health system?" So we started saying," Well, can we help identify patients who maybe have COVID that are maybe spreading it to others unknowingly? Maybe we can identify them ahead of time." So I reached out to Steve's team and they said," Yeah, let us help." Steve's team is really good at geo mapping and high level details at the block level of patients, and so we started talking. They had a feed of patients who had COVID in the community. At the time, this was late March, early April. There were just a handful of cases. So we said," Well, if someone has COVID and they live within a quarter mile of that person, maybe they have been exposed. Let's alert the health system. If that person has an appointment in the next two days and they're coming into the hospital or the clinic and they've been around someone else with COVID, let's create a system where we can operationalize screening them ahead of time." So Steve's team created this list. They passed it straight into our EHR, into an in- basket pool that the nurses would then work, and we would do direct outreach on the patients. Obviously, a quarter mile was kind of ridiculous, and that quickly changed to about a four- block radius. And then Steve's team did what they do really well, is they created a very sophisticated and very accurate tool for estimating prediction of being in high proximity to someone with COVID.
Paul Anderson: And then Dr. Miff, is that what we're talking about when we're talking about the proximity index score? Is that what we're talking about there?
Steve Miff: Right. Paul, right from the beginning, one of the first things that we knew was that proximity mattered, right? We also knew that to be able to effectively manage and help Parkland, the county, the community management, we needed to take a hyper localized approach to our data. So as Dr. Moran said, from even before we saw significant cases in our community, we're able to expand our data relationship with the county to be able to get daily feeds of the testing data to know exactly who is testing positive. And by doing that and taking those cases, those individuals, geo mapping them so we knew exactly where they're in the community, we can take any individual-- In this case, we started with the Parkland patients, as Dr. Moran said, that were scheduled for an appointment within the next two days-- and dynamically use these analytics to calculate a risk, a proximity COVID risk for themselves. Those that were identified as having high risk because of not only their proximity to positive active cases but also the density of those cases, they would be teed up within the electronic medical record for proactive outreach. Our intent was if somebody has a high risk of having been infected, let's not bring them in into environment where they can spread it to others, but we still wanted to meet their clinical needs. Hence, the transition of those to virtual visits. So that was a very practical way of adjusting at the individual level and also providing options on what's the best way to care for them. That was sort of the start of leveraging a proximity index that relied on these algorithms without needing to actually directly track somebody's movement themselves or having them download another app. Because where apps were emerging in the marketplace that we're really tracking, this is where it really gets into sort of the equity component of that. A lot of the patients that Parkland was serving might not have either the technology or the smartphones to do that, or might not have the digital, technical knowledge to do that or use them effectively. So how do we help them in a way that's less intrusive and it's equitable across the board?
Paul Anderson: Well, that points to a limitation of using those apps in that way, right? They rely on every individual participant to take the action. And anytime you've got a gap there, you're going to have a gap in the incoming data and you're going to have a little bit less complete picture. Yeah. I imagine that once you've got this proximity index and you have started to apply it, that other applications of the index would make themselves clear. Did you find that to be the case? And if so, what types of other uses?
Brett Moran: Exactly, Paul. First of all, we deployed this and the nurses, PCCI would send in. It was every three days they'd send in a new batch of people that had appointments in the next two days that had a high proximity risk, and then we would call them and we would ask them two questions. Do you have any COVID- like symptoms? Have you been around anyone who has COVID? So with that first initiative, we then tracked the data and it was interesting. When we asked people if they had COVID- like symptoms who had a high proximity risk identified by PCCI, 18% endorsed those symptoms. When we asked them if they had been near someone, very close to someone who had COVID, those people who had been indicated as high proximity risk, 26% endorsed positive symptoms. And then if we took the people who were flagged as high proximity risk by PCCI and we actually tested them, 24% were positive for COVID, so one in four. So it was actually a very accurate tool, and it was very helpful. So for the first initiative trying to protect, looking inward, people coming into the health system... We don't want them to spread it to other patients. We don't want them to spread it to other staff. It was highly effective. We screened over 230,000 patients, over 1. 2 million encounters. We found this to be a very useful tool, and it helped. It kind of decrease the spread within our health system. But then as you said, Paul, the question was, what else can we do? Steve and his team and I met, and we said," Well, let's look outward. Let's look at people who don't have an appointment in the next 30 days, but who maybe live in a high risk area, a high proximity risk." And then we said," Well, we really don't have enough nurses to call." Because that was over 400,000 people. So we said," Well, let's do two things. Number one, let's automate this. So let's not call them. Let's send them a text message with a link to a survey." So we used an automated survey tool, and they would fill that out rather than us doing that manually. And then we also said," If a person is young and healthy and has high risk for COVID, they're generally going to do very well. So the bang for the buck, there's not as much value in screening them, but let's focus on the people that have high vulnerability." So we again turned to PCCI and we said," Let's try to create a tool that can estimate vulnerability, and then let's screen these people. And let's not only screen them for risk of COVID, but if they have high risk, let's go ahead and ask them if they have medical needs, and we can offer to them so they don't need to come in. We can get them prescriptions. We can do a virtual visit. And let's also ask them if they have food insecurity." Because food insecurity was something that was really coming out as the biggest social determinant that came out in the pandemic. There were just millions of people around who were having a lot of food insecurity in the state. So we would ask them if they needed food, how many people? We partnered with a food pantry that would deliver them groceries for 14 days, which was enough time to let them clear if they had COVID so they didn't need to go out and get any food. That was kind of the second part of this proximity initiative. But again, it was key on Steve and his team developing this vulnerability risk score.
Paul Anderson: You described it would be impractical to have nurses actually physically pick up the phone and call every single person you were screening, because there's hundreds of thousands. But does that act of pushing out the outreach and then tacking onto that, as you said, some of these other services that you might be offer, is that one of the ways that this ties into fighting inequitable access to care and really reaching out to people who might otherwise not encounter the healthcare system for whatever reason?
Brett Moran: Yeah. It's kind of silly to say, but people don't like talking to people. People like texting. So if we could have a solution that used text, and they could use their phone, and they could click a link and fill out the survey. We had very good response to that, and we had surprisingly high percentage of people that endorsed food insecurity. So it did work, and maybe not as well as trying to call people directly. Although, people don't generally pick up the phones. But it helped when we needed to mass scale this kind of thing.
Steve Miff: And Paul, what I would add is that to address inequities, it's a multidimensional effort, and it absolutely starts with the type of data and the type of analytics that you use to make sure that you actually look at the whole person, the whole community and not introduce artificially things that downstream will have an impact. So in this case with the vulnerability analysis and the index, we took multiple metrics and multiple data, and brought it together to be able to again, look comprehensively at an individual. We knew from the beginning that age was a risk factor. We knew that there were specific medical comorbidities that were associated with high risk of COVID. Certainly, those were important to include. But right at the heels of that, we also intuitively knew from the work that we've been doing in this community for years and years, that the ethnic backgrounds, the racial components as well as the information about somebody's life, the things that we call the social determinants of health likely are a key component that also contribute to that COVID risk. So we group those and look at those as factors that do not change rapidly in the short term but are critical, and then pair those up with two additional factors, one being around mobility. We knew that the more folks move around, whether it's by necessity or by choice, that would make them and those round the more vulnerable. We also knew from the first wave that proximity matters, so the density of active cases. We took these multiple components together and built this algorithm to be able to identify the vulnerability, both at the individual level and then look across the geography. So the activities and the urgency of those activities were prioritized based on where were the folks that were most vulnerable and also where you had the highest density of cases across the community. So whether it's the mobile testing sites or new testing sites that are being stood up, they're done in a way that folks across the community can access them conveniently on their own term. That's where I believe that starting with information that takes into account those key elements is so critical, because then any action that you start to take downstream, if you don't do it right the front end, it's already being biased.
Paul Anderson: Well, and if I understand right, you touched on some of the activities you were able to help coordinate in the community. But did that then extend to, once the vaccine started to become available, to advising for instance the county on setting up vaccination locations, right? Because there's this whole conversation about," Well, we got to get everybody, but we can't do everybody in the first day. How do we distribute that?" Was that contributed there as well?
Steve Miff: Paul, you're spot on. It's interesting, right? With each new wave of the pandemic, new challenges were being created. And when vaccines first became available in December of 2020 and then more widely available in January, that was a key component because we had more demand for vaccines initially than we had supply. So a key analytical exercise, and then all the activities to follow were," Okay, so how do we prioritize? Who should get that vaccine first?" One of the other, I think, really great things that occurred across Dallas was the coordination between Parkland, other healthcare providers, the county, and the city to create one central registration list. Everybody work off the same list, pair that up with, again, the data that we've talked about, and then ongoing with the vaccination data so we knew on a daily basis who received their vaccine. We needed to take those lists of hundreds of thousands of individuals and prioritize in a way that's most equitable, and we get to the folks that need it the most first. So that's where the two analytics that we historically used on both vulnerability and the proximity came together, enabled us to actually prioritize daily those lists based on those factors that we just talked about, and start to send the registration messages and work down the list in that order. So then, somebody who could get to the internet first or somebody who could register 20 times did not get prioritized versus somebody that was much more vulnerable from both their ethnic, racial, their socioeconomic, their medical conditions, et cetera. That's something that at the beginning we used and in a very effective way to prioritize and get those vaccines to individuals.
Brett Moran: Yeah. I think, Paul, as Steve said, we were very intentional. We knew this was coming. The CDC talked about this at the first wave. There would be much more demand than there would be vaccinations, and so we wanted to be very intentional about being equitable with the vaccine distribution. So whereas everybody had to follow the 1b list, which we all did, we prioritized our 1b list based on vulnerability, and those were the first people. At Parkland, we sent them a ticket to schedule, and only people with a ticket could schedule their appointment. We started with the most vulnerable and worked our way down the list. All of the 1b ultimately received a ticket, but it was the prioritization of those that were most vulnerable who also happened to be those that had the most socioeconomic disparity. So it was very intentional, and we would call them. We would send them text messages and emails. We let them schedule online. We had call centers calling them. And the results held true. At Parkland, of our vaccines we distributed, 49% went to Hispanic, 23% went to Black, 18% to white. And when you compare that with the Dallas County race ethnicity makeup, it's very favorable. 40% of Dallas is Hispanic. We've vaccinated 49%. 23% is Black, we vaccinated 23%. So it was very useful. Looking at social determinants, over 50% of patients who received vaccines lived within the highest socioeconomically inequitable zip codes, and so it was very fortuitous. We were very lucky and we were very happy with the results that it ended up being an equitable distribution.
Paul Anderson: Well, and when you compare that with what Dr. Miff was describing earlier about people not having the same access to smartphones for instance, and you think about the experience around the country. You'd hear about people waiting up till midnight, because that's when the pharmacies list refreshed and they could try to get an appointment first thing, or doing that on behalf of their grandparents or something like that. This seems like not only a better way to ensure equity, but also a better way to ensure... How do I want to say it? Some rationality to the process.
Brett Moran: Yeah. No. Agreed.
Steve Miff: And Paul, I'll give you also one example, because I think we oftentimes learn from things that we look back and say," Boy we could have done that better." By actually using data daily and updating this, you actually have the opportunity to learn and adjust quickly. Dr. Moran talked about the 1b and that component of how things and, I would say, the upfront filters that were coming as guidance from the federal, from the state governments of how you prioritize. One of those first groups was really good rationale when you look at it. It was let's prioritize folks that were older, over the age of 75 as the first category, and then take that group and use the method that we said. But we quickly realized that applying that upfront filter, you automatically introduce an equality component to it. We knew that the zip codes that Parkland serves, you move a couple miles and the life expectancy drops by 15 years. You had communities where the average life expectancy is 63. Well, those also in some cases the folks that needed it the most, but by applying well- intentioned, a filter that let us focus on those that are over the ag of 75, you could automatically introduce something that's not favorable. So by tracking the data and looking at this every day, you're able to pivot really quickly. But again, I think it makes the point. You need to think from the first step all the way through, and make sure that every step along the way you don't unintentionally introduce something that could create an inequity in the way you approach.
Paul Anderson: I always like to wrap up these conversations by asking for something that people who are listening can do today to advance equity and safety. And recognizing they're not going to be able to do everything all at once, they need a starting point. One of the things I particularly liked that I think we can really learn from your experience is that while you certainly had an infrastructure in place to gather this data, you weren't looking for this data specifically, I'm going to guess, before the pandemic was on all of our minds. So you really were, in some cases, really starting up. Dr. Moran, maybe I'll start with you. If another organization is looking to develop or assess their community equity programs and how they interact with their community in this way, what's a good starting point?
Brett Moran: Paul, I think I would suggest start small. One of the mistakes that I think I've made and I think a lot of people make is you try to swallow the whole elephant. I would pick one, no more than three areas that you want to focus on, and then do your equity spin looking at real data, race, ethnicity, and language. For me, I find frequently the best way to move the needle is just to make the data available. Not to make a metric out of it, not to make it punitive. Just to make it available. And when people see it, they start changing practices. A lot of the things that we described today, we had data points. We would show the proximity index. We put it in our EHR, which is a standard bread and butter EHR that many, many people use, and we put it in the schedule so you'd see it when the patient showed up. We put it in our alerts. We put it in our all of our standard system lists, our patient lists. We made it easy to find this information so that it could be applied into the everyday, because that's what you want them to do, is to embed it into their everyday practice. So take a few small metrics and then embed them, and hopefully the needle will start to move.
Paul Anderson: And Dr. Miff, any thoughts from you?
Steve Miff: Yeah. Paul, to me there are two key things. One, leverage the data that you have to its full extent. We're not experiencing a lack of data. It's just we're experiencing a lack of practical application of the data. What we've seen, that folks can start today on their journey, is take the information they already have on your patients from your electronic medical record and enrich it with the information that you already have about where they live. It's a fairly straightforward process of geo mapping your patients so you understand where they live, and then attribute those factors that are in publicly available data in a lot of cases so you better understand the situations that they're living in. That provides you so much more perspective into what barriers they have to access your health system, whether it's physical access or whether it's digital access as new organizations are starting to embark on that. You can proactively address those. Because without them, we can create access points that might be inequitable or not everybody can get to in a way that's convenient or accessible to them. That's one. We have the information. It's just a matter of bringing it together. And the second one, Dr. Moran also alluded to. Make it visible and accessible within where people already do their work. Don't expect them to hear something great and then expect them to go somewhere else to find it. If you're not teeing it up exactly where they're already doing the work in a way that's easily digestible, it's not going to have its biggest impact. So small steps, but towards this goal of knowing your patients better, and understanding them better leads rapidly to such great improvements anywhere from strategy, from access, as well as to the frontline point of care interventions.
Paul Anderson: Okay. Before we wrap up, is there anything that either one of you wanted to add before we wrap it up?
Brett Moran: Well, I think, Paul, Steve alluded to the next. I think some people may be saying," Well, what do you do next?" For Parkland, beginning with using COVID predictions and doing some of the modeling behaviors and things that led us to saying," What do we do with big data? Can we get big data to help better inform us and tell us about who are our patients?" So as Steve mentioned, we want to know who are they, patients? What's important about our patients? We know their medical problems. We know some of these things. We're starting to touch the surface of social determinants of health, but we're really not getting into the bigger... There's a lot of environmental and social data on our patients that are outside of the EHR that we don't know about, but it is there, and it is readily available information. Now that we all have complex health systems and big computer systems and data analysis, you can actually create an amalgam of that data to get you a better 3D picture of the patient and who they are. Those are some things we're working with Steve and his team on. We call it the Know Thy Patient Initiative, and it's telling us more about who they are. Because again, with healthcare, the outcomes, 80% of it is not related to the care they receive. It's related to the environment and the genetics and the habits, and we need to know more about that to reflect their care and provide equitable and good care for them.
Paul Anderson: Dr. Moran, Dr. Miff, thank you so much for joining us. You can learn more about ECRI and the ISNP PSO from the equity website at www. ecri.org where you'll find more conversations in this podcast series about racial and ethnic disparities in care. You can find more about Parkland Center for Clinical Innovation at pccinnovation. org and Parkland Medical Center at parklandhospital. com. Be sure to subscribe to Smart Healthcare Safety on Spotify, iTunes, Google Play, or wherever you get your podcasts to get our latest episodes. We welcome your feedback. Please visit us at ecri. org or email us at ecri- podcast @ ecri. org.
DESCRIPTION
This episode is part of a series regarding the ECRI and the ISMP Patient Safety Organization's (PSO) annual Deep Dive report.
The 2021 Deep Dive report focuses on issues of racial and ethnic disparities in healthcare. Research has repeatedly confirmed that members of racial and ethnic minority groups are more likely to experience disparities in care, including having an increased risk of being uninsured or underinsured, lacking access to care, and experiencing worse health outcomes for treatable and preventable conditions.
In this episode, our guests discuss their data-driven approach to identify and combat disparities and realize their vision of creating a Dallas County with equitable, accessible healthcare for all. Our first guest, Brett Moran, MD, is from Parkland Health & Hospital System, one of the country’s largest and most progressive safety-net hospitals. Our second guest, Steve Miff, PhD, is from Parkland Center for Clinical Innovation, a leading, non-profit, data science, artificial intelligence and innovation organization affiliated with Parkland Health & Hospital System.
Visit ECRI and the ISMP PSO to learn more or to request a demo. Download the executive brief of ECRI and the ISMP PSO's Deep Dive, Racial and Ethnic Disparities in Health and Healthcare today.
Today's Host
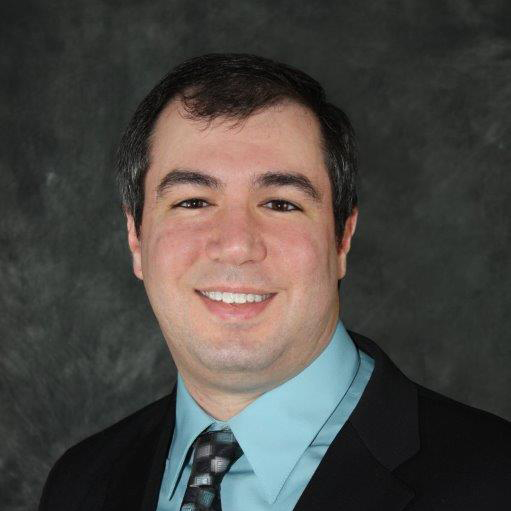
Paul Anderson
Today's Guests
Steve Miff, PhD, MS
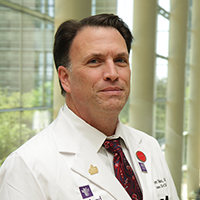